
Researchers at Rice University have developed a new machine learning (ML) algorithm that excels at interpreting the “light signatures,” or optical spectra, of molecules, materials and disease biomarkers, potentially enabling faster and more precise medical diagnoses and sample analysis.
“Imagine being able to detect early signs of diseases like Alzheimer’s or COVID-19 just by shining a light on a drop of fluid or a tissue sample,” said Ziyang Wang, an electrical and computer engineering doctoral student at Rice who is a first author on a study published in ACS Nano. “Our work makes this possible by teaching computers how to better ‘read’ the signal of light scattered from tiny molecules.”
Every material or molecule interacts with light in a unique way, producing a distinct pattern ⎯ like a fingerprint. Optical spectroscopy, which entails shining a laser on a material to observe how light interacts with it, is widely used in chemistry, materials science and medicine. However, interpreting spectral data can be difficult and time consuming, especially when differences between samples are subtle. The new algorithm called Peak-Sensitive Elastic-net Logistic Regression, or PSE-LR, is specially designed to analyze light-based data.
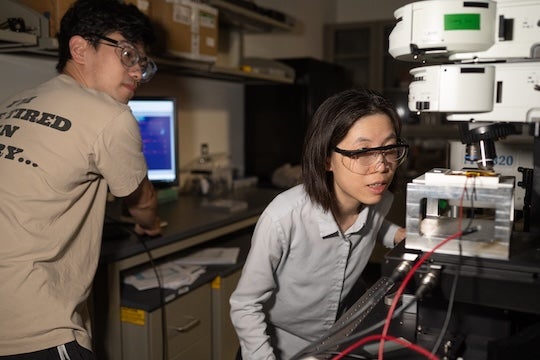
“The optical spectra of a tissue or other biological sample can reveal a lot about what’s happening inside the body,” Wang said. “This is important because faster and more accurate disease detection can lead to better treatments and save lives. Beyond health, our method can also help scientists understand new materials, leading to smarter sensors and smaller diagnostic devices.”
PSE-LR can not only accurately classify different samples but is also transparent in its decision-making ⎯ something that many advanced ML models are not particularly good at. PSE-LR delivers a “feature importance map” that highlights exactly which parts of the spectrum contributed to a classification decision, making results easier to interpret, verify and act on.
“Our algorithm was designed to focus on the most important parts of the signal ⎯ the peaks that matter most,” Wang said, comparing PSE-LR to “a detective learning to find clues hidden in light signals.”
The researchers tested PSE-LR against other ML models, showing improved performance especially in identifying subtle or overlapping spectral features.
“Most models either miss the tiny details or are too complex to understand,” Wang said. “We aimed to fix that by building something both smart and explainable.”
The model also performed well in a range of tests gauging its real-world acumen, including detecting ultralow concentrations of the SARS-CoV-2 spike protein in fluid samples, identifying neuroprotective solutions in mouse brain tissue, classifying Alzheimer’s disease samples and distinguishing between 2D semiconductors.
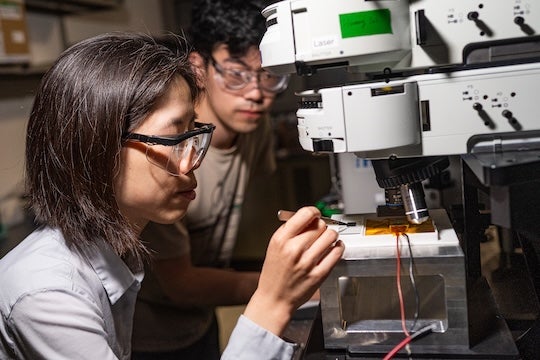
“Our tool is able to parse light-based data for very subtle signals that are usually hard to pick up on using traditional methods,” said Shengxi Huang, an associate professor of electrical and computer engineering and materials science and nanoengineering who is a corresponding author on the study.
The new algorithm could enable the development of new diagnostics, biosensors or nanodevices.
“These findings could help transform medical diagnostics and materials science, bringing us closer to a world where smart technologies help detect and respond to health problems faster and more effectively,” Wang said.
The research was supported by the National Science Foundation (2246564, 1934977), the National Institutes of Health (AG077016-02) and the Welch Foundation (C-2144). The content herein is solely the responsibility of the authors and does not necessarily represent the official views of the funders.
- Peer-reviewed paper:
-
Machine Learning Interpretation of Optical Spectroscopy Using Peak-Sensitive Logistic Regression | ACSNano | DOI: 10.1021/acsnano.4c16037
Authors: Ziyang Wang, Jeewan C. Ranasinghe, Wenjing Wu, Dennis C.Y. Chan, Ashley Gomm, Rudolph E. Tanzi, Can Zhang, Nanyin Zhang, Genevera I. Allen and Shengxi Huang
https://doi.org/10.1021/acsnano.4c16037